Limitations of qualitative data
Unlike quantitative data, which is often readily available in spreadsheets, qualitative data tend to lack an easily defined structure that facilitates data analysis. In addition, interpreting non-numerical data can be challenging, while clear formulas exist that researchers can follow to compare quantitative values. Moreover, in semi-structured interviews or focus groups, researchers may ask follow-up questions that can't easily be predicted. An interesting answer may lead to deeper questions to search for more in-depth insights.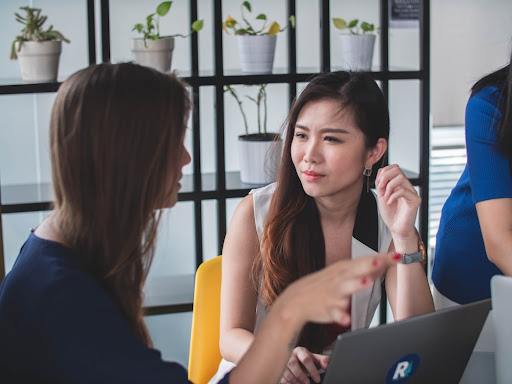
The need for the interviewer to pursue deeper answers can impede the organization of data into neat rows and columns. However, it is important to organize the data so that different meanings that emerged across participants or data sources can be assessed. Researchers often need to take time to reorganize their data to facilitate interpretation.
Moreover, interpreting non-numerical data is a significant challenge for qualitative researchers. The relative quantitative value of different things can be relatively easy to interpret.
An example
If someone takes the temperature of New York and the temperature of Chicago on the same day and gets two different values, asserting that one city is warmer than the other would be uncontroversial. After all, one need only get a numerical value representing the temperature in each city to come to a fairly straightforward conclusion.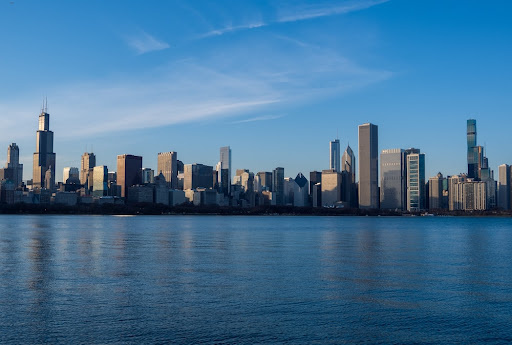
However, people may disagree about what makes a city interesting or exciting. To take from our example about music, people may even disagree about whether the visual or performative elements of music should be considered. Thus, the researcher needs to clarify the potential differences in understanding between people.
Analyzing qualitative data to answer such research questions requires transparency in analysis. Researchers analyzing socially constructed, subjective concepts should clearly define their concepts so their audiences understand the data analysis.